Consonant effects on F0 in Chinese
Language Log 2014-06-12
Following up on two earlier Breakfast Experiments™ ("Consonant effects on F0 of following vowels", 6/5/2014; "Consonant effects on F0 are multiplicative", 6/6/2014), here are some semi-comparable measurements of consonant effects on fundamental frequency (F0) in Mandarin Chinese broadcast news speech.
[As I warned potential readers of those earlier posts, this is considerably more wonkish than most LLOG offerings.]
Why do people care about the effects of consonant features on F0? The main reason is that tonogenesis — the historical development of lexical tones – often arises from re-interpretation of "micromelodies" of this kind, typically driven by laryngeal features of consonants such as voiceless vs. voiced (e.g. p,t,k,s vs. b,d,g,z). So it's natural to wonder whether languages where this has already happened, like Mandarin Chinese, retain or suppress such effects.
According to one line of argument, the consonant-induced micromelodies are a natural and more-or-less inevitable consequence of the fact that voice pitch and consonant voicing are implemented by the same laryngeal apparatus, so that F0 differences will always accompany voiced/voiceless distinctions. If some of these tonal differences have been re-interpreted as the primary or only features distinguishing words, with the original consonantal distinctions being lost, this won't have any effect on the consonants of retained or new consonant voicing distinctions.
Another line of argument points out that the historical process that transforms segmental micromelodies into lexical tone must involve an exaggeration of the segmental effects at the same time as the loss of the original consonantal cause. And if the effects can be exaggerated (or at least implemented independent of the original consonantal distinction), maybe they're not so automatic after all, and can be attenuated as well, so as not to confuse the cues for tonal distinctions.
The data for today's experiment came from a collection of 1997 Mandarin Broadcast News Speech (LDC98S73) published by the Linguistic Data Consortium in 1998. The selection process is described as follows, in Jiahong Yuan, Neville Ryant, and Mark Liberman, "Automatic Phonetic Segmentation in Mandarin Chinese: Boundary Models, Glottal Features and Tone", ICASSP 2014:
We extracted the “utterances” (the between-pause units that are time-stamped in the transcripts) from the corpus and listened to all utterances to exclude those with background noise and music. Utterances from speakers whose names were not tagged in the corpus or from speakers with accented speech were also excluded. The final dataset consisted of 7,849 utterances from 20 speakers.
This same collection was used in two recent papers on automatic tone classification: Neville Ryant, Jiahong Yuan, and Mark Liberman, "Mandarin Tone Classification Without Pitch Tracking", ICASSP 2014; and Neville Ryant, Malcolm Slaney, Mark Liberman, Elizabeth Sriberg, and Jiahong Yuan, "Highly Accurate Mandarin Tone Classification In the Absence of Pitch Information", Speech Prosody 2014. The dataset (as used in the three cited papers) is in the queue to be published by the LDC later this year.
In this experiment, I used the training-set part of this collection, comprising 7,549 utterances and 6.05 hours of speech. The translation from Hanzi (Chinese-character) transcripts to pinyin with tone was done automatically for this material, as was the alignment of the tone-pinyin transcription with the audio. Checking against a test corpus of 300 hand-transcribed and hand-aligned utterances, we found 93.1% agreement of alignment within 30 msec., and 1.2% wrong assignment of tones.
As a reminder, here are average F0 contours for the four main Mandarin Chinese tones, taken from 7,992 syllables produced in sentence context from 8 speakers — the data comes from Jiahong Yuan's PhD dissertation ("Intonation in Mandarin Chinese: Acoustics, perception, and computational modeling", Cornell University 2004).
For exploring consonant effects on F0 in Mandarin, I've broken things out by tone as well as by consonant — tones 1, 2, 3, 4 in syllables whose initial consonants are /k/ vs. /g/, /t/ vs. /d/, /p/ vs. /b/, /s/ vs. /z/. As I did in the case of the English data, I've taken the first 50 msec. of F0 estimates from each vowel — a sequence of 10 estimates – and scaled those ten sequential estimates by their average value. Thus a value of 1.05 for the first F0 estimate in a particular vowel means that the value at that point was 5% greater than the average of the values over the first 50 msec. of that vowel. I then averaged these vectors of scaled estimates, element-wise, for all tone-1 syllables beginning with /k/, all tone-2 syllables beginning with /k/, and so forth.
Thus from the start of one particular vowel (in the syllable ke2) we get the sequence of F0 estimates (rounding to one decimal place)
156.2 150.5 148.5 149.6 135 136.7 138.6 139.6 140.3 141.7
These were calculated with a spacing of 5 msec., so the 10 estimates span 50 msec. altogether.
The mean value is 143.7, and so the scaled values are
1.087 1.047 1.033 1.041 0.94 0.952 0.965 0.971 0.977 0.986
My script repeats that calculation for all 69 instances of tone-2 syllables whose initial is /k/, and averages element-wise, resulting in the sequence
1.068 1.046 1.025 1.011 0.995 0.983 0.975 0.973 0.976 0.979
which is what is graphed using the plotting symbol "k" in the graph in the second column of the first row of the array below:

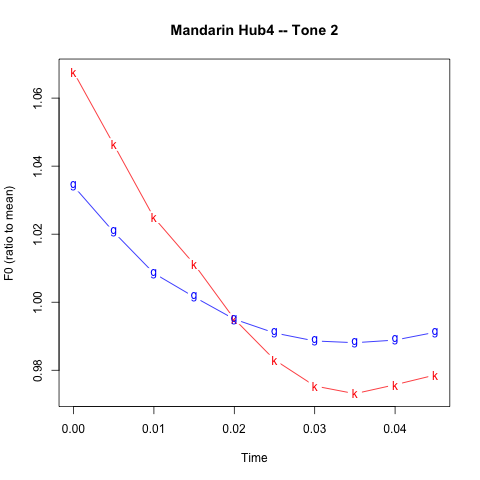
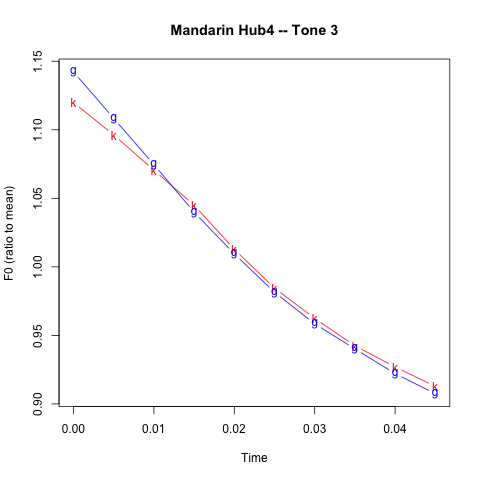
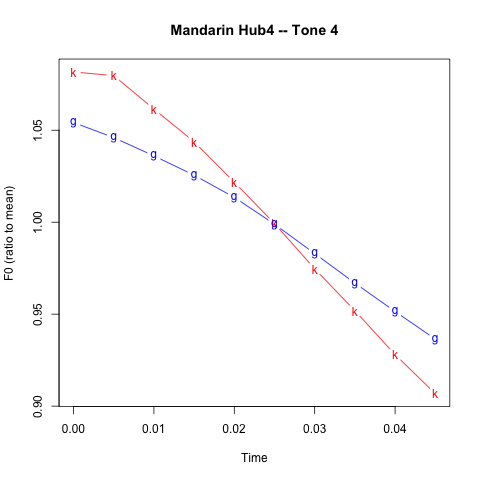

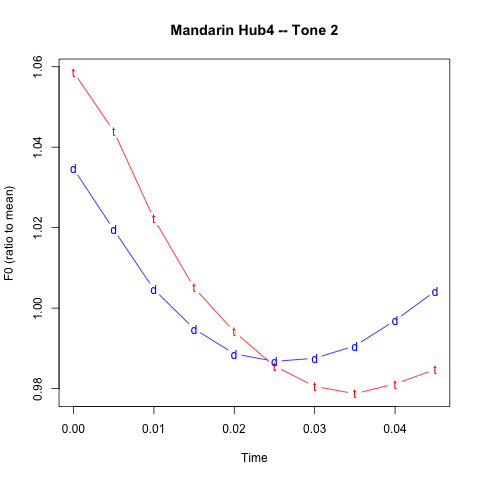
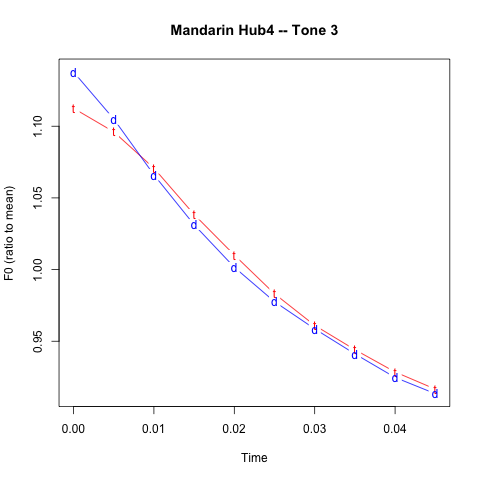
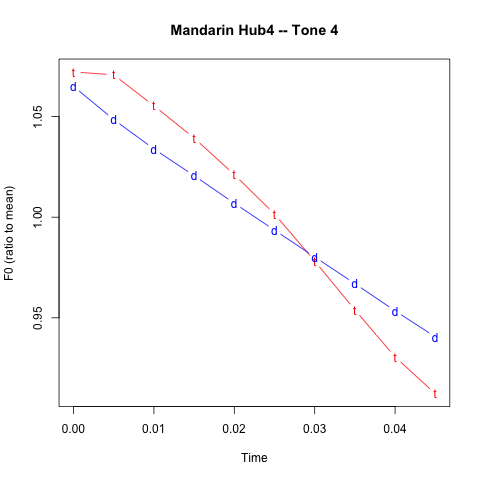

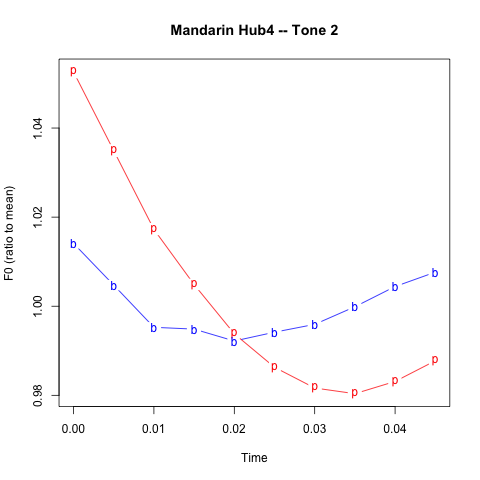


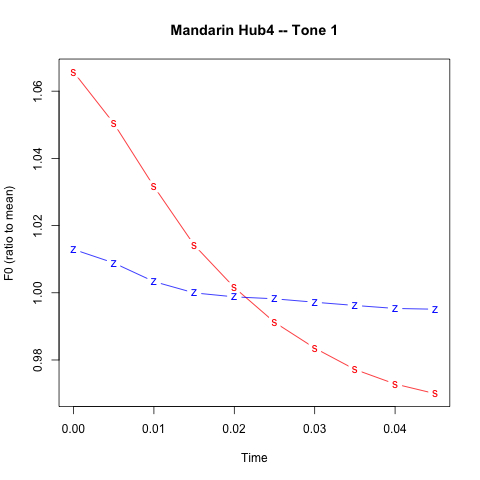
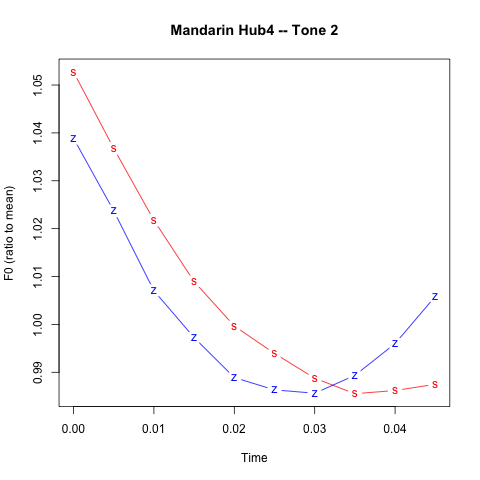
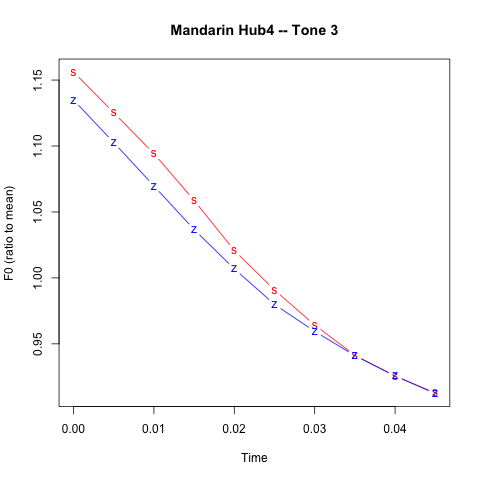
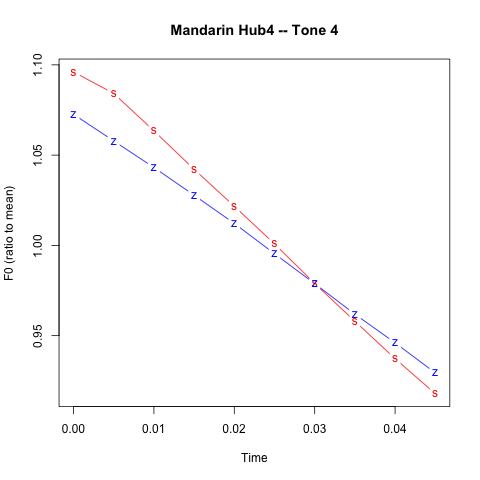
In the case of tone 1, the difference between voiced and voiceless initials seems very similar, both qualitatively and quantitatively, to the difference in English, as shown in the previous posts:
The other tones look somewhat different, and in particular, the difference seems to be almost entirely gone in tone 3, and rather attenuated in tone 4. Why? I don't know — it's worth further investigation, I think.
And that investigation will have to give up on the simple graphical averaging technique I've used so far, and find a way to use regression to explore the relationship between the many relevant factors and the F0 contours of individual syllable onsets.
In particular, the nature of language entails that real-life usage is highly non-orthogonal in various ways, which mere dataset-size doesn't change. Thus (for example) the set of 69 tone-2 syllables starting with /k/ in the Chinese Broadcast News dataset I used were
55 k e2 6 k uang2 4 k ao2 1 k ui2 1 k u2 1 k an2 1 k un2
where I would guess that "ke2" was mostly 可 ke3 "can; may; able to; certain(ly); to suit; (particle used for emphasis)" after 3rd-tone sandhi. The 2,317 instances of tone-2 syllables starting with /g/ were distributed as
2155 g uo2 108 g e2 25 g uan2 11 g ang2 6 g an2 6 g u2 6 g uang2
where again I would guess that "guo2" was mostly 国 guo2 "country; state; nation".
Particular words — e.g. "can, may, be able to" vs. "country, state, nation" — will have particular distributions of phrasal position, emphasis, etc., which will effect pitch range, vocal effort, etc. A larger dataset will just bring these highly-skewed distributions into better alignment with the overall average for (some particular variety of) the language in question.
Also, the consonantal feature of "voicing" has more than two values, both phonologically (where both voiced and voiceless stops can be aspirated or not, for example) and phonetically (where e.g. English "voiceless" stops have widely varying degrees of relative timing between laryngeal and oral articulations). And it's also worth noting that different genres and styles of speech — here TIMIT sentences read by volunteers in an acoustics laboratory vs. news stories presented on the air by professional announcers — are likely to be different in various ways.
To make sure that the effects we see are the effects we're looking for, and not the result of some correlated variable(s), we need to consider and evaluate many alternative hypotheses.
So on balance I think it's amazing that I got any interpretable results at all.