The Affordances of Content Design
e-Literate 2019-09-18
Content is infrastructure.
David Wiley
I opened my first post in this series with a statement about courseware and content design:
An unbelievable number of words have been written about the technology affordances of courseware—progress indicators, nudges, analytics, adaptive algorithms, and so on. But what seems to have gone completely unnoticed in all this analysis is that the quiet revolution in the design of educational content that makes all of these affordances possible. It is invisible to professional course designers because it is like the air they breathe. They take it for granted, and nobody outside of their domain asks them what they're doing or why. It's invisible to everybody else because nobody talks about it. We are distracted by the technology bells and whistle. But make no mistake: There would be no fancy courseware technology without this change in content design. It is the key to everything. Once you understand it, suddenly the technology possibilities and limitations become much clearer.
That's all true. But this series isn't really about courseware. It's about the capabilities and limitations of digital curricular materials, whether they are products sold by vendors, OER, or faculty-developed. The content design pattern I'm exploring is neither unique to vended courseware products nor invented by commercial courseware providers. In fact, instructional designers and LMS providers have been desperately trying to convince faculty of the value of this course design pattern for a many years. But designing content this way takes a lot of work and lacking good examples of the return on that investment, most instructors have not opted to build their content this way.
What the proliferation of commercial courseware provides that is new is a wealth of professionally developed examples that we can examine to better understand how this content design pattern works to support certain teaching and learning affordances in digital curricular materials. In this post and the next, I will draw on some of those examples, which happen to come from Empirical Educator Project sponsors, to show the design pattern in action.
The most important message of this series, for both educators and technologists, is that real advances in educational technology will almost always arise out of and be best understood through our knowledge of teaching and learning. In this case, technological affordances such as learning analytics and adaptive learning are only possible because of the instructional design of the content upon which they operate. And we sometimes forget that "instructional design" means design of instruction. The baseline we are working from is instructional content, generally (but not exclusively) designed for self-study. How much value can students get from it? How far can we push that envelope? Whatever the fancy algorithms may be doing, they are doing it with, to, and around the content. The content is the infrastructure. So if you can develop a rich understanding of the value, uses, and limitations of the content, then you can understand the value, uses, and limitations of the both technologies applied to the content and the pedagogical strategies that the combination of content and technologies afford.
The role of digital curricular materials
Let's start by looking at the holistic role that digital curricular materials play when implemented in a way that the design pattern supports. From there, we'll back into some of the details.
I'm going to ask you to watch a short promotional video from Pearson of a psychology professor who participated in one of their efficacy studies shares here experiences and observations about teaching with their courseware products. (You should know that Pearson has engaged me as a consultant to review their efficacy reports, including this one, to provide them with feedback on how to make those reports more useful.) The fact that this professor's story is part of a larger efficacy study means that it is richly documented is ways that are useful to our current purpose.
As you watch, pay attention to Dr. Williamson says about the affordances of the content and how those affordances support her pedagogical strategies and objectives:
Dr. Manda Williamson of University of Nebraska-Lincoln on her courseware experimentThe first thing she talks about is layered formative assessments. Students are given small chunks of content followed by frequent learning activities. They then are prompted to take formative assessments which, depending on the results and the students' confidence levels, may result in recommending additional activity. (The one mentioned in the video was "rereading.") If your anchor point for the value of the product is the readings that you assign for homework, then you can see how interactive content that is well designed in this way might be an improvement over flat, non-interactive readings (or even videos).
When the students come into class—and this is key—Dr. Williamson engages with them on the results of their formative assessments. She teaches to where the students are, and she knows where they are because she has the data from the formative assessments.
How does that work?
Those assessment items are tied to learning objectives. Skills and knowledge that have been clearly articulated. In well designed content, the learning objectives have been articulated first and the assessment questions have been written specifically to align with those learning goals. With this content design work in place, creating a "dashboard" is not technologically complicated or fancy at all. No clever algorithms are necessary.
Suppose you give students five questions for each learning objective. One way you could create a dashboard is to show a line item for each learning objective and show what percentage of the class got all five questions right, what percentage got four out of five, and so on. I'll show some example dashboards from other products later in this post. For now, the take-away is that the students are basically taking low-stakes quizzes along with their readings, and the instructor is getting the quiz results before the class starts so that she can teach the students to where they are.
Hopefully the formative assessments don't feel like "quizzes;" Dr. Williamson has positioned them as tools to help the students learn, which is how exactly how formative assessments should be positioned. But the main point is that the content includes some assessed activity which enables the teacher to have a clearer understanding of what the students know and what kinds of help they may need.
As a result of adopting the digital content design and teaching strategies that the content and technology affordances supported, Dr. Williamson's DFW rate dropped from 44% to 12%. Since her course is a gateway course, that number is particularly important for overall student success. So it's a dramatic success story. But it's not magic. If you understand teaching, and if you look at the improvements made in the self-study content and the in-class teaching strategies, you quickly come to see that it's not technology magic but thoughtful curriculum design, solid product usability and utility, and hard work in the classroom that produced these gains. Technology played a critical but highly circumscribed supporting role.
You can read more about Pearson's efficacy study, ranging from an academic account of the research to a more layperson-oriented educator guide, here.
Design details
It might help to make this a little more concrete. I'm going to provide a few example screens in this post that are fairly closely tied to the basic affordances that I've discussed above, and then I'm going to explore some more complex variations in the next post in this series.
I mentioned earlier that the formative assessments should function like quizzes but that students should not feel like they are being tested. This idea—that the assessments are to help the students rather than to examine or surveil them—is built into the design of good curricular materials in this style. For example, Lumen Learning's Waymaker courses of a module that explicitly addresses this idea with the students:
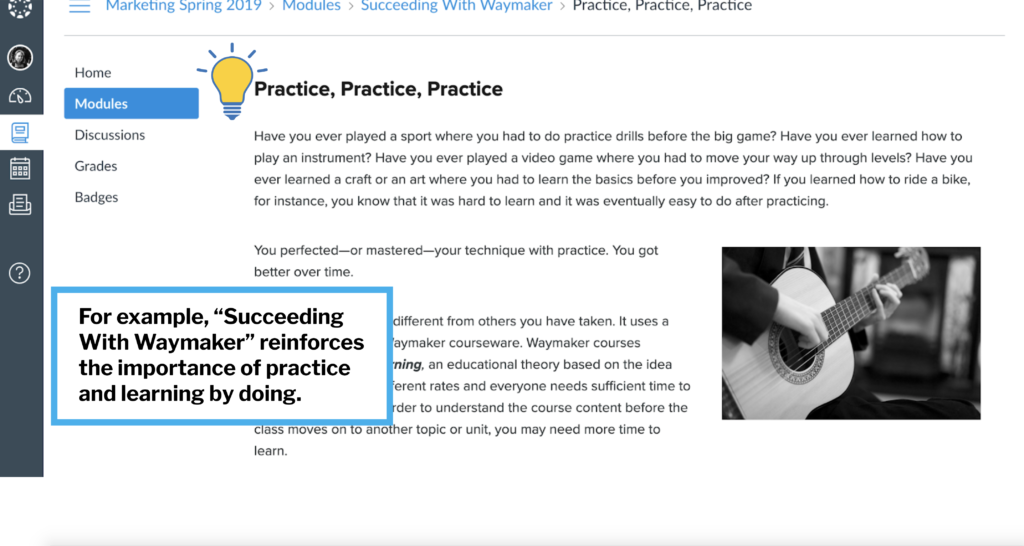
The Waymaker product then uses the formative assessments the students take, tied to their learning objectives, to show students the associated content areas where they have shown mastery and others where they still need some work. This student dashboard is called the "study plan":
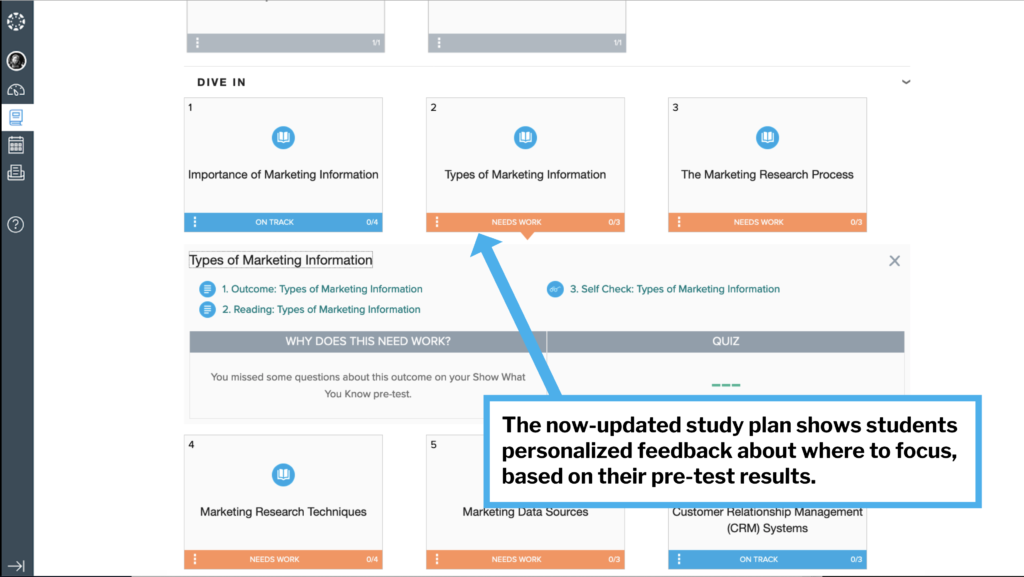
Lumen Learning's study plan updates based on formative assessment scores.
There are different philosophies about how to provide this kind of feedback. One product designer told me one philosophy he was thinking about is that the best dashboard is no dashboard, meaning that giving student little progress indicators and nudges are better. For educators evaluating different ways to deliver the content, the commonalities provide the tools for evaluating the differences. "Data" are (primarily) the formative student assessment answers. "Analytics" are ways of summing up or extracting insights from the collection of answers, either for an individual student or for a class. "Dashboards," "nudges," and "progress indicators" are methods of communicating useful insights in ways that encourage productive action, either on the part of the student or the educator.
Speaking of the latter, let's look at some educator dashboards. Let's look at a dashboard from Soomo Learning's Webtext platform. Even before you get into how students are performing on their formative assessments, you might want to know how far students have gotten on their assigned work. This might be particularly important in an asynchronous online course or other environment where you have particular reason to expect that students will be moving along at different paces. So this dashboard sorts student by their progress in a chapter:
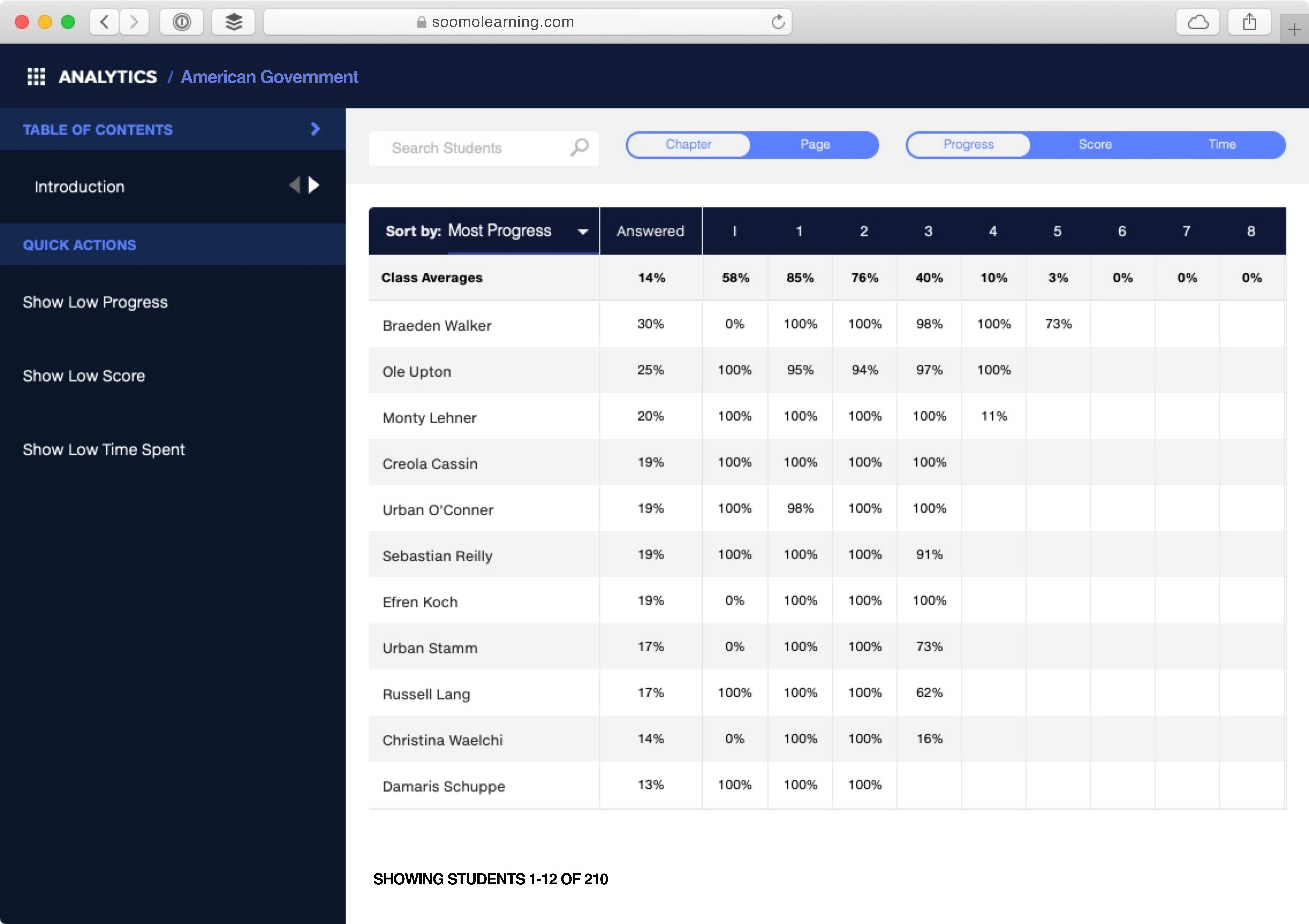
Notice that progress here is measured by percentage of questions answered. That tells us something about where the product designers think the value is. A formative assessment isn't only a measure of learning progress. It is also a learning activity in and of itself. We learn by doing. We learn more effectively by doing and getting instant feedback. So rather than measure pages viewed or time-on-page (although we do see a toggle option for "time" in the upper right-hand corner), the first measure in the dashboard is percentage of questions answered.
Drilling down, Soomo also shows percentage correct by page:
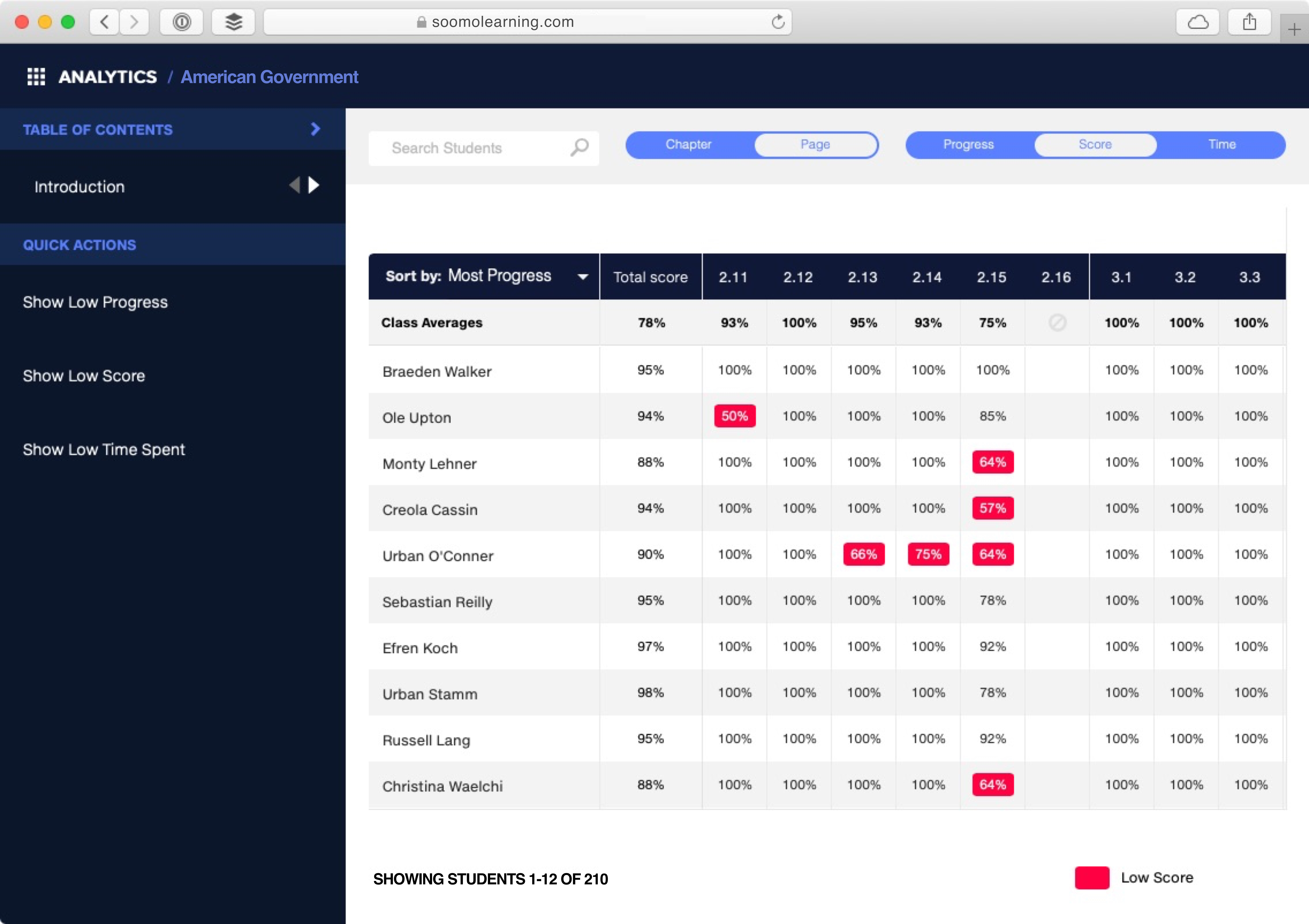
There's a bit of a rabbit hole that I'm going to point to but avoid going down regarding how cleanly one can separate learning objectives. Does it always make the most sense to present one and only one learning objective per page? And if so, then what's the best way to present analytics? Rather than explore Soomo's particular philosophy on that fine point, let's focus on highlights of the low scores. This is one detail that instructors will want to know at some fairly fine level of granularity. (If two learning objectives are on the same well-designed page, it's usually because they're closely related.) This dashboard enables instructors to see which students, both individually and as a group, scored poorly on particular assessments on a page.
Again, there's no algorithmic magic here. Let's assume for the sake of argument that the content and assessments are well designed. Soomo is thinking about what educators would need to know about how students are progressing through the self-study content in order to make good instructional decisions. They are then designing their screens to make that information available at a glance.
Now imagine for a moment that you have this kind of increased visibility on how students are doing with their self-study. You see that students are doing well on a learning objective overall, but they're struggling with one particular question. In the old world of analog homework, you might not catch this sort of thing until a high-stakes test. But with digital curricular materials, where you can give more formative assessment and have it scored for you (within the bounds of what machines are capable of scoring), you might quickly find one particular problem in an assessment that students are struggling with. Is the question poorly written? Is it catching a hidden skill, or a twist that you didn't realize made the problem difficult? You'd want to drill down. Here's a drill-down screen from Macmillan's Achieve formative assessment product:
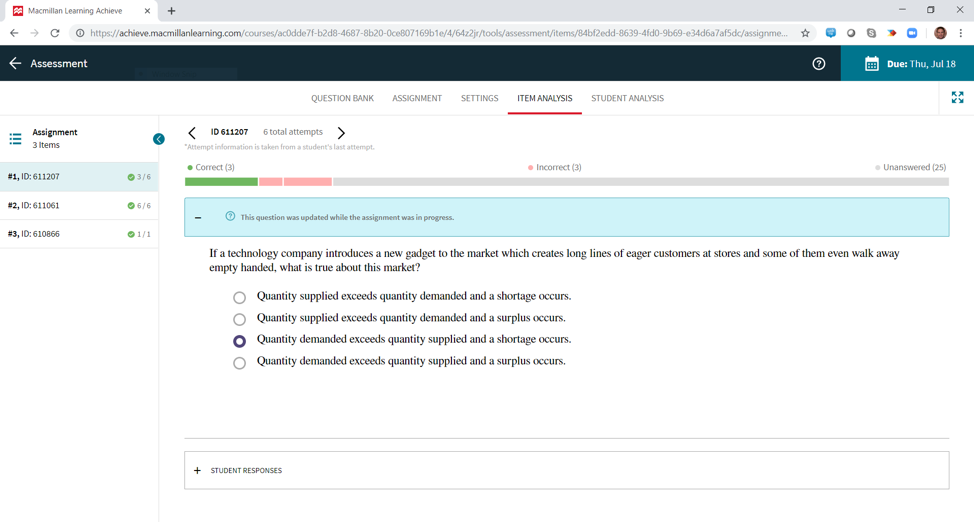
(You should know that I serve on Macmillan's Impact Research Advisory Council.)
This is exactly the sort of clue that an educator might want to look at while preparing for a class. What are the unusual patterns of student performance? What might that tell us about hidden learning challenges and opportunities? And what might it tell us about our course design?
Hints of what's coming
I'll share two more screen shots as a way of teasing some of the concepts coming in the next post. This first one is from Carnegie Mellon University's OLI platform:
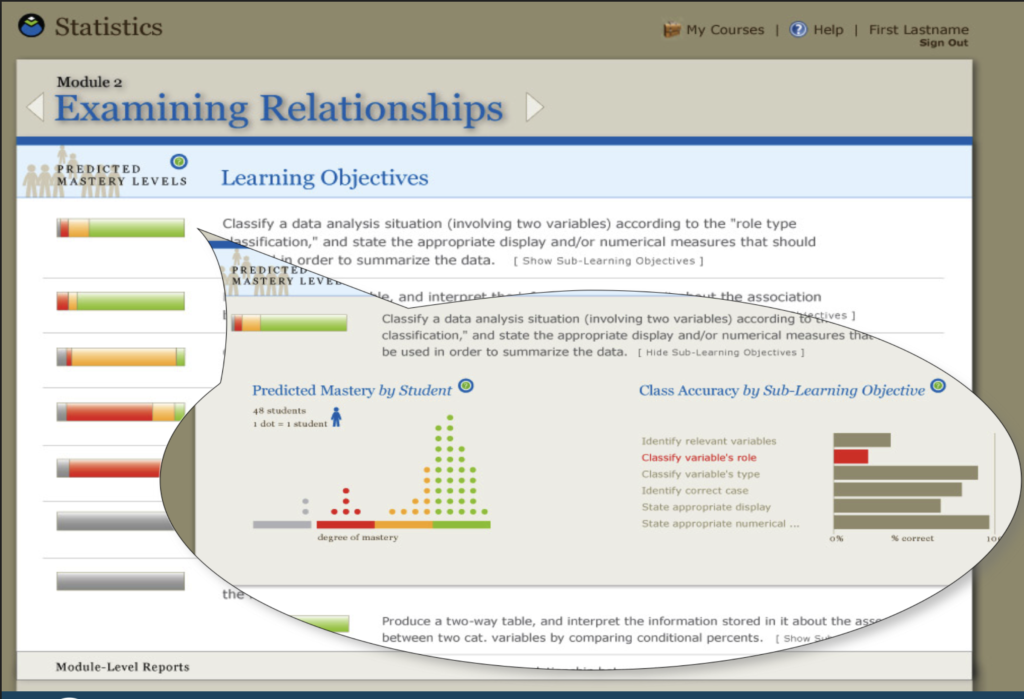
At first glance, this looks like another learning dashboard. What percentage of the class are green, yellow, or red (or haven't started) for each learning objective? But notice one little word: "predicted mastery levels." Predicted. Once you start collecting enough data, by which we mean enough student scores to begin to see meaningful patterns, we can apply statistical analysis to make predictions. There is a certain amount of justifiable anxiety about using predictive algorithms in education, but the problem springs from applying the math without understanding it. That's what predictive algorithms are, at their most basic. They're statistical math formulas. And honestly, many of the predictive algorithms used in ed tech are, in fact, basic enough that educators can get the gist of them. We've been taught to believe that the magic is in the algorithm. But really, most of the time, the magic is in the content design.
And here's a screen from D2L Brightspace:
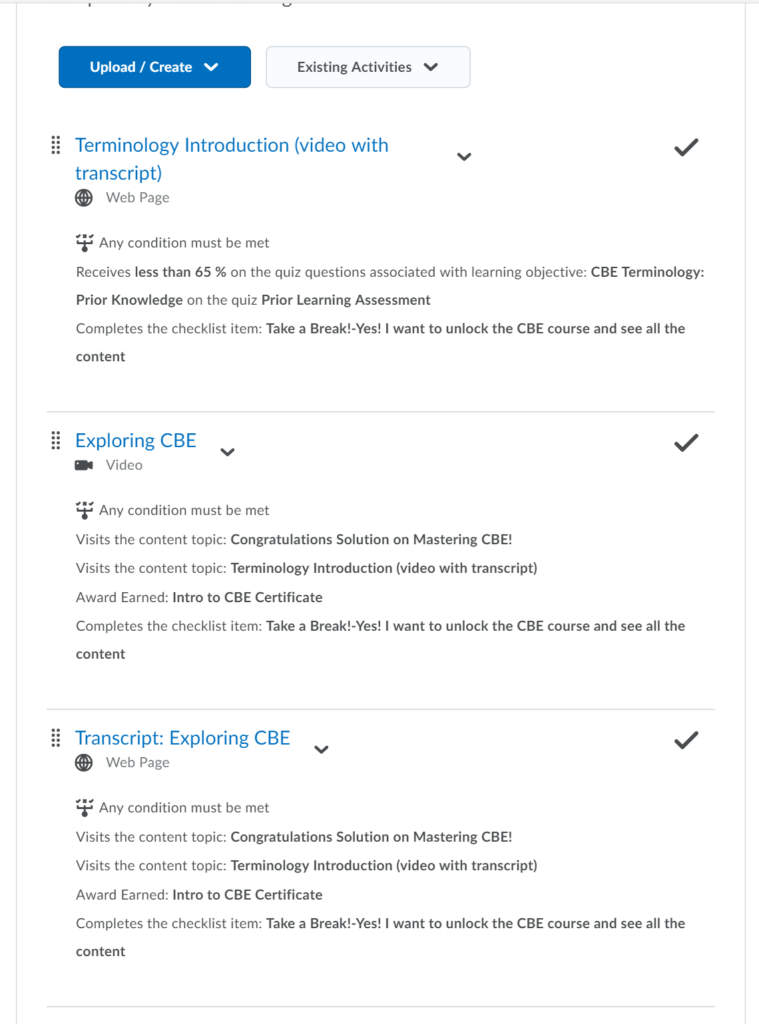
There's a lot to unpack here, and I won't be able to get to it all in this post. This is a tablet view of functions that Brightspace has been building up for ever and day. Since long before modern courseware existed as a product category. For starters, you can see in the top box that Brightspace can assign mastery for a learning objective. (In this case, the objective happens to be "CBE Terminology: Prior Knowledge.") But what follows is a set of simple programming instructions of the form, "If a student meets condition X [e.g., receives less than 65% on a particular assessment] then perform action Y [e.g., show video Z]." In the olden days of personal computers, we would call this a "macro." In the olden days of LMSs, we would call it "conditional release." Today's hot lingo for it is "adaptive learning" or "personalized learning." Notice in this example that we are still starting with performance against a learning objective. We are still starting with content design.
(Note also that many of the technology affordances built into vended courseware are also available in content-agnostic products like LMSs and have been for quite some time. Instructors can build content in this design pattern with the tools they have at hand and gain benefits from it.)
In the next post, I'm going to talk about how advanced statistical techniques, including machine learning techniques, and automation, including what we commonly refer to as adaptive learning, are methods that digital course content designers use to enhance the value of their course content designs. But all of those enhancements still build off of and depend upon that bedrock content design pattern that I described in the first post of this series.
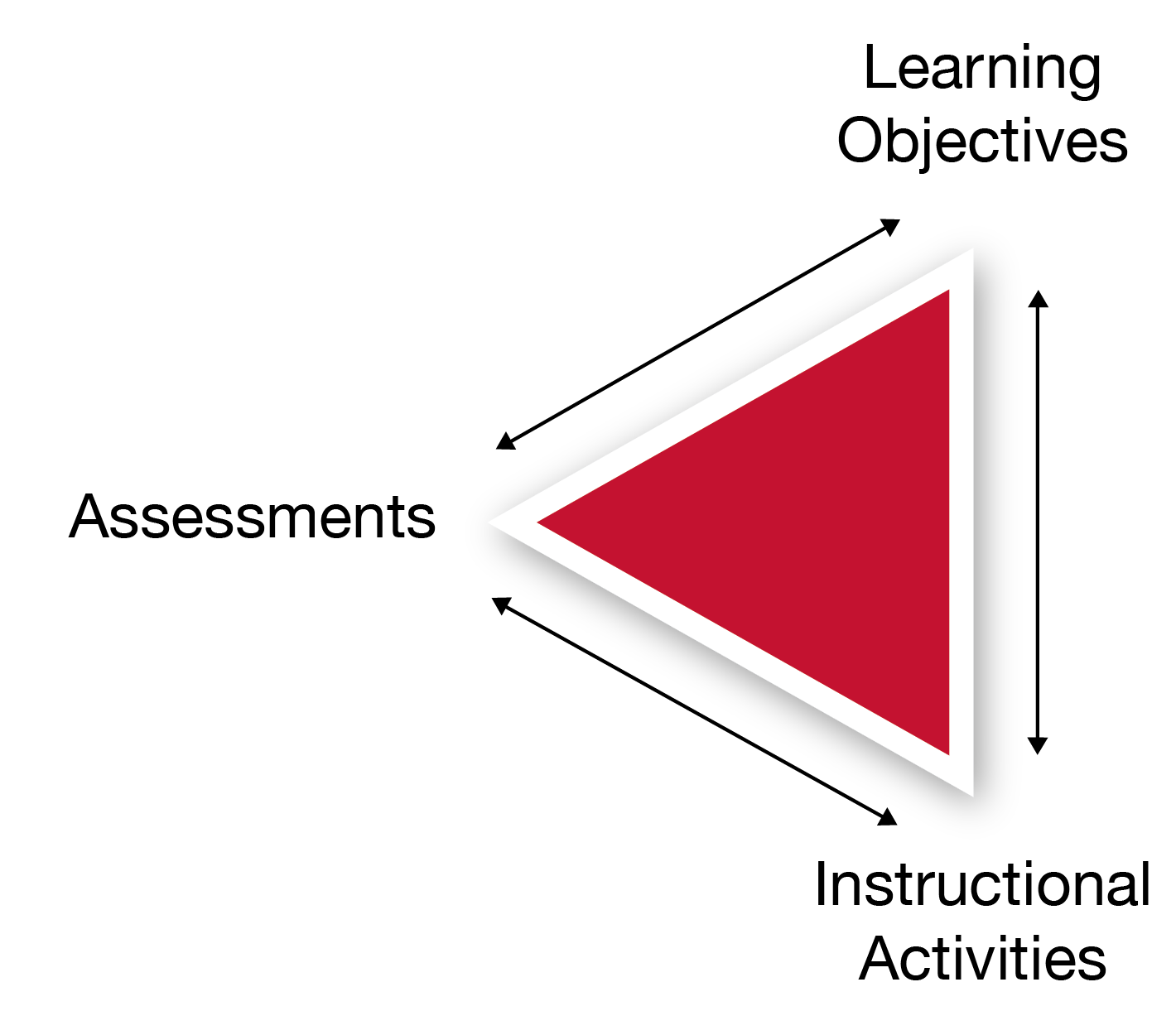
The atomic unit of digital curricular materials design
The post The Affordances of Content Design appeared first on e-Literate.