The Anatomy of a Scaled Digital Seminar
e-Literate 2020-11-10
In my last post, I argued that scaling the seminar is an equity issue:
[S]caling the seminar is essential for equity if we want to avoid a two-track system of education—one at expensive universities and another for the rest of us. This is already a serious problem that the current situation is likely to make worse.
Scaling the Seminar
I also argued that we are experiencing a failure of imagination about how to scale the seminar because EdTech has become obsessed with solving the wrong problem:
We’ve been pursuing the solutions that have helped improve student self-study by providing machine-generated feedback on formative assessments. While these solutions have proven beneficial in some disciplines and have been especially important in helping students through developmental math, they have hit a wall. Even in the subjects where they work well, these solutions usually have sharply limited value by themselves. They work best when the educators assigning these products use their feedback to reduce (and customize) their lectures while increasing class discussion and project work.
Despite the proliferation of these products, flipped classrooms and other active learning techniques are spreading slowly. And meanwhile, there is a whole swath of disciplines for which machine grading simply doesn’t work. This most broadly applies to any class in which the evaluation of what students say and write is a major part of the teaching process. EdTech has been trying for too long to slam its square peg into a round hole.
Scaling the Seminar
In this post, I will outline an approach for scaling social feedback and peer assessment as the means for scaling the seminar. I will use the example of an English composition course for two reasons. First, I recently had the pleasure of presenting at a virtual workshop on the topic hosted by the University System of Georgia and supported by the APLU. So I have already done some thinking about this particular topic. Second, English composition is one of the hardest seminar-style classes to scale. Students need frequent and intensive feedback. And particularly in access-oriented contexts, many of these first-year students come to college with the skill sets necessary to provide high-quality writing-oriented peer review. So it’s a good test case.
Setting the goalposts
Let’s be clear about the boundary between ambitions and fantasies. I do not believe it is currently possible to scale English composition courses with quality in even middle-of-the-road access-oriented colleges using any method that I can think of. We do not have a viable alternative to frequent feedback from an expert human to teach students that don’t have a good grasp of grammar, are weak at constructing arguments, don’t know how to cite evidence, and have not learned how to give and take feedback on writing.
I do believe that a technology-supported approach to social feedback and peer assessment can potentially accomplish the following goals:
- Improve the outcomes in access-oriented composition courses by supporting effective pedagogical models and evidence-based practices
- Make writing-across-the-curriculum programs more practically implementable.
- Scale effective seminar-style classes in cases where students are better prepared for them (including second-year students at access-oriented institutions who have had a year of experience in a writing-across-the-curriculum program).
- Provide scaffolding for faculty in all disciplines to “flip” their classes more easily and effectively
The basic rationale is that social feedback and peer assessment enable groups to learn and work more effectively regardless of the knowledge domain. These two functions are at the heart of seminar-style pedagogy. Anyone lucky enough to have taken a great seminar on any subject will likely remember the fantastic conversations among the whole class. There may or may not have been lectures. But whenever content is provided to the students—as an instructor presentation, a reading, or something else—the point is generally to catalyze peer-to-peer conversation and learning.
Equally importantly, these skills transfer to the workplace. With all the buzz over the years about preparing students for the future of work, we fail to recognize that the future of knowledge work—a future that has already arrived—is a workplace environment designed to facilitate social feedback and peer assessment in a project-based learning context. For example, in software development, Agile software development is all about improving software quality by baking social feedback and peer assessment into the day-to-day work of building software. The very first tenet of the Agile Manifesto—which only has four tenets in total—is “individuals and interactions over process and tools.” Building novel and complex software always requires a learning process. It’s a major reason why development timelines are notoriously unpredictable. Teams learn as they build. They discover unanticipated problems to which they have to invent solutions. Older software development methods perpetually led to disappointment because they failed to recognize that knowledge work entails learning and discovery. Waterfall development was optimized for predictable schedules. It failed at that goal. In contrast, Agile software optimizes for team learning and responsiveness in the face of unpredictability. It has a much better track record.
Today, Agile software development is used to create and improve many of the largest and most complex software applications in human history. These projects succeed by deploying—and continuously fine-tuning—social feedback and peer assessment at scale in a project learning-based context. The rule of thumb with Agile software development teams is that they are usually seven people on average. Larger projects are scaled by creating more teams, not larger ones. The various Agile methodologies all emphasize team roles, regular check-ins, and frequent constructive feedback from teammates. Agile development is organized in sprints, which are short periods of time—typically two weeks—in which teams attempt to meet very specific project goals that were defined at the beginning of the sprint. (You can think of them as project-based learning objectives.) At the end of each sprint, teams conduct “demos” to bring in important stakeholders and get peer review on what they accomplished. They also conduct “retrospectives,” in which the team discusses what went well and what went poorly in each sprint, with a goal of identifying ways to improve their craft in future sprints. They might conduct formal exercises like “the five whys” which are designed to improve the quality of social feedback.
Agile can teach us a lot about what it takes to improve both the quality and the scale of purposeful collaborative learning work. Experienced practitioners will tell you that Agile is easy to explain but hard to do well. Managers of development shops often need years of practice and regular coaching from experts to cultivate high-performing collaborative teams reliably. And importantly, many Agile teams do use specialized tools to support their collaborative learning journey. The Agile Manifesto may prioritize individuals and interactions over processes and tools, but most Agilistas are all for processes and tools designed to support and scaffold individuals and interactions.
You may even be familiar with one or two of these tools. Trello—a collaborative brainstorming and planning tool with a whole section of its website devoted to educators—was originally developed as an Agile software development tool to simulate sticky notes moved around on a whiteboard with swim lanes drawn on it. It is owned by Atlassian, which is probably the single best-known company for creating products designed to support Agile software development.
I’m not arguing that every class should ape Agile development methodologies. It’s the opposite, actually. Every Agile development methodology is an example of a project-based collaborative learning methodology. While we don’t need to fetishize software development, we certainly can learn from it. The first two lessons are (1) collaborative, active learning can be accomplished effectively at scale, and (2) doing so is hard, requiring lots of practice for everyone and good supporting tools.
Individuals and interactions over process and tools
That last bit—about the approach being hard and requiring lots of practice for everyone—is a buzzkill. The popular assumption is that that that neither professors nor students will work hard and persistently, especially if it requires them to change their habits. Rather than interrogating that assumption, the sector gets seduced into magical thinking. “Humans are too hard to teach. Why don’t we teach machines to do the work instead? That will be much easier!” Which, I must say, is not a terribly productive attitude for education in general. But it’s particularly bad for teaching difficult and uniquely human skills like good communication and critical thinking. Let’s take a moment to explore that dead end before moving on to the approach I’m proposing.
As part of my preparation for the aforementioned workshop on scaling English composition, I took a look at the notes the group had been taking from previous sessions. They (inevitably) included a tools section that (inevitably) included a list of programs that auto-assess writing. To be clear, I use tools like these in my own writing. But I am vehemently opposed to their use in education as unsupervised assessment tools. These programs all regularly provide mistaken diagnoses and poor advice. I am an experienced enough writer to know when to ignore them. Many students are not. The students who are in most dire need of frequent feedback on their writing are also least well equipped to know when to ignore the software’s advice. And the software is often poorly equipped to handle second language learners or neurodivergent students with unusual language or communication challenges.
To illustrate this point, I tested a few text examples with Hemingway, one of the tools listed in the workshop notes. For my first test, I asked Hemingway what it thinks of a sentence that Noam Chomsky famously created to illustrate the difference between syntax and semantics.
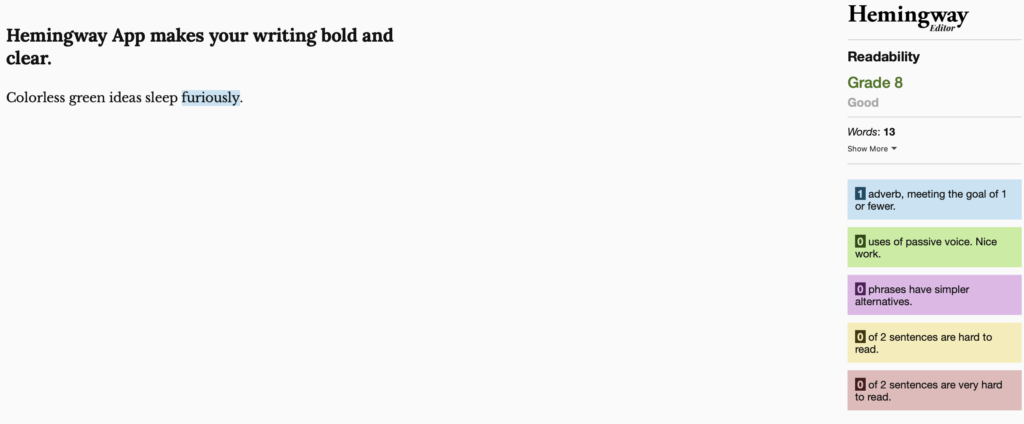
This sentence is perfectly grammatical and perfectly meaningless. Hemingway likes it.
Maybe that’s an unfair test. Let’s try some text from Hemingway’s fellow giant of American literature and notorious macho man, Herman Melville:
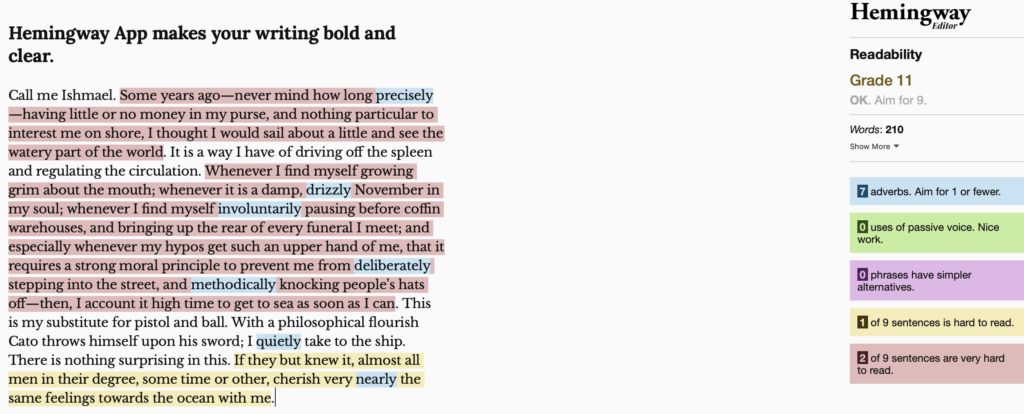
Hemingway likes “Call me Ishmael.” After the first three words, though, things go downhill fast.
So, maybe that’s not fair either. Let’s try some non-fiction. How about a great speech?
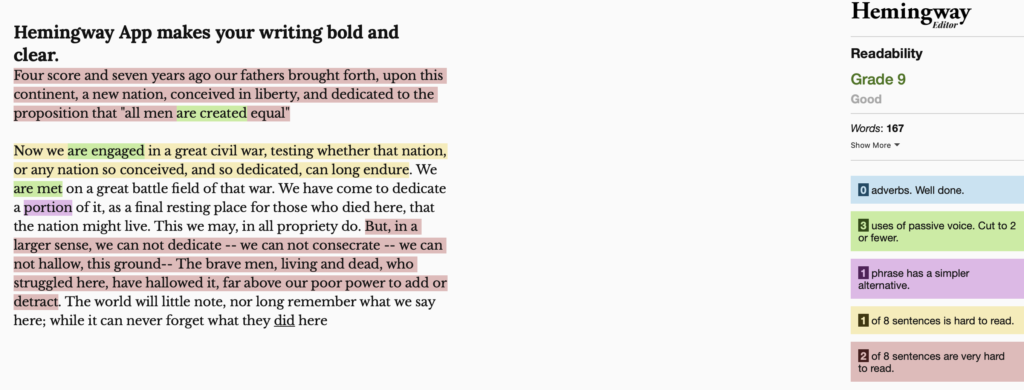
Hemingway is not a big fan of Abraham Lincoln either, as it turns out. It thinks the Gettysburg Address is “very hard to read.” And maybe that’s true. But I don’t think that Lincoln would have written a better speech had he followed Hemingway’s advice.
At this point in my experimentation, I had given up on finding another great American writer that Hemingway likes. So just for fun, I threw it a Robert Frost poem:
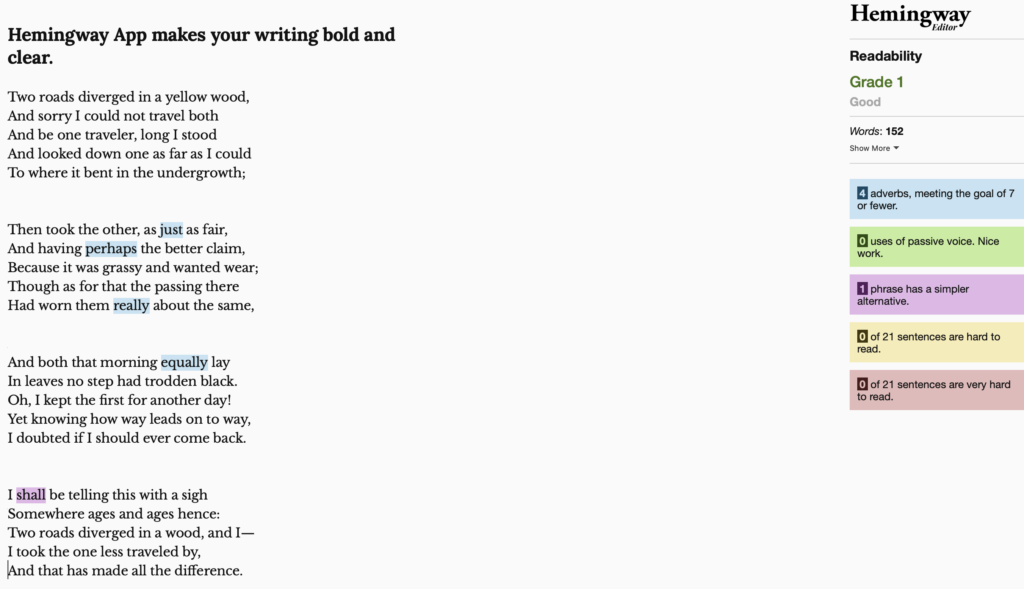
Huh. Hemingway was not thrown off by the line breaks of the poetry. Does it “understand” them? Or does it fail to “see” them at all? I don’t know.
And this is the real problem with the machine assessment of writing. It’s not just that the software gets the analysis wrong a lot. That’s bad enough. But the bigger problem is that it gets its analysis inscrutably wrong a lot. How do you teach students when to trust an unreliable tool if you don’t know when it will be reliable yourself? The more advanced a textual analysis algorithm is, the greater the chances are that even the people who programmed it don’t always know why it makes the assessments that it does. This is because artificial intelligence software reprograms itself as it “learns” in ways that even AI experts can’t always follow.
Machines are unpredictably unreliable writing tutors. And keep in mind that Hemingway is only trying to evaluate writing style here. Software that tries to evaluate the accuracy or coherence of a written argument is even more problematic.
If your software cannot evaluate complex speech acts, it is probably useless at evaluating many types of complex thought. And isn’t complex thinking…um…what we’re supposed to be learning how to do in college? Might that be one reason that the seminar is considered the pinnacle of a high-quality college education?
Collaborative feedback
Throughout this post, I have been using the phrases “collaborative feedback” and “peer evaluations.” I consider them to be separate competencies, with collaborative feedback being a prerequisite of peer evaluation. These skills are building blocks for any kind of social learning pedagogy, including but not limited to the seminar.
Collaborative feedback is essentially class discussion that actively managed by an educator (or facilitator if the exercise is in the workplace). Participants both practice both giving and receiving constructive feedback. In contrast to other styles of class discussion, collaborative feedback sessions are student-centric. The goal of the instructor is to encourage peer-to-peer conversation and then intervene primarily to help keep the participants on track and constructive. The the group has achieved competence when the instructor either starts to function as just another peer in the group or fades out of the conversation altogether.
This is a common strategy for teaching English composition. By way of example, let’s revisit the Hemingway evaluation of the Gettysburg Address:
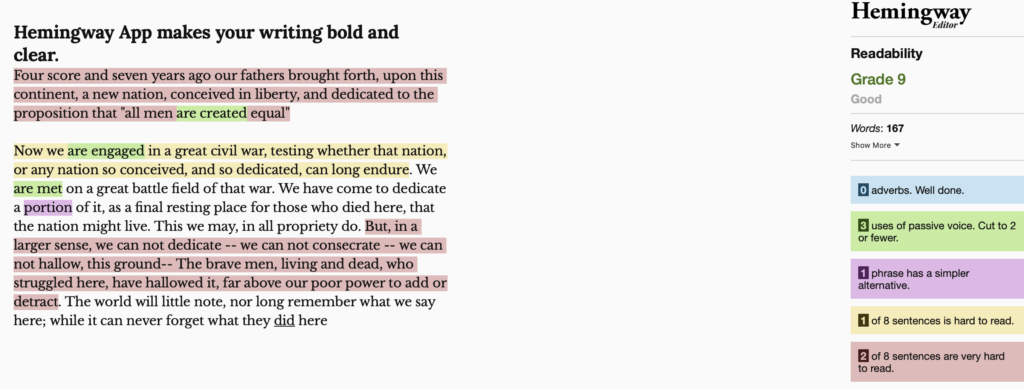
Students achieve competency at writing collaborative feedback when they can have a constructive conversation about the writing passage without a lot of help. This takes time and practice. The instructor might start by modeling commentary. What does that first sentence mean? What would be a simpler way of writing it? Why did Lincoln write it that way instead of putting it more simply?
Students are learning a whole cluster of skills in this exercise, including but not limited to the following:
- How to ask good questions about writing quality (starting with, “Do I actually understand this sentence?”)
- What intelligent answers to those questions look like
- What constructive answers for the purposes of revision look like
Writing instructors do this all the time. Sometimes they use passages from readings, while other times they use student writing samples. They don’t need a tool like Hemingway to do this work. But notice how it can help. It scaffolds some of the questions and calls students’ attention to sentences that deserve attention. With an educator present to facilitate, Hemingway could be an excellent aid for fostering collaborative conversation around writing. (If I were facilitating such a conversation using this passage in Hemingway, I might point out that one of the uses of passive voice is contained in a quote from the Declaration of Independence. So there’s an opportunity to teach students the skill of knowing how to use tools like Hemingway and when to ignore them.)
In collaborative feedback sessions, participants are always learning and refining two sets of skills: one that is domain-specific and another that is general to collaborative work. It is not enough for participants’ answers to be insightful. They must also be constructive. Instructors are often scaffolding both of these skill sets. First, they model. Then, they facilitate. And finally, they step back and let the students lead.
Scaling an access-oriented English composition seminar is incredibly difficult because it often takes an entire term to get students proficient enough at collaborative feedback that they will be ready for peer evaluation, which is the point at which social teaching methods can scale. (In fact, access-oriented writing classes in challenging environments routinely fail to get all the students across this particular finish line. It’s too much to accomplish in one course.)
But I can easily see how both synchronous and asynchronous technologies could enable instructors to teach collaborative feedback skills more effectively. The Hemingway example I just gave is a good starting place. A good textual analysis tool could help the instructor rapidly scaffold many different examples for class discussion. The Riff Analytics tool that I wrote about in my Engageli first look post is another example. Riff may not be able to evaluate the quality of the student feedback, but it can provide feedback on the collaborative conversation quality. Some education-oriented discussion boards are starting to develop interesting affordances that also help students to cultivate this skillset. For example, Ment.io provides analysis of how close or far apart students are from each other in their opinions on a subject, based on textual analysis:
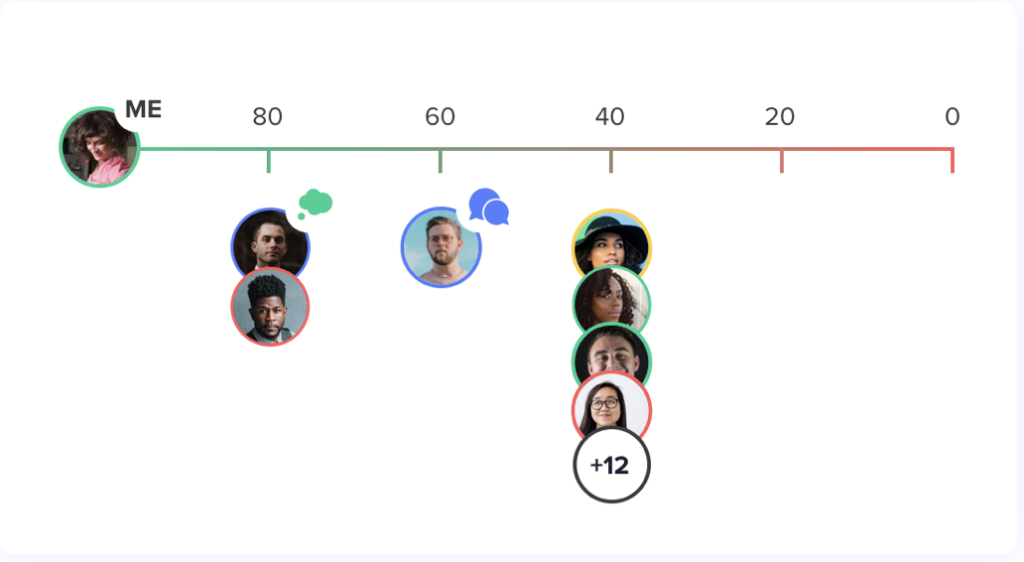
How accurate is this algorithm? I don’t know. But it’s fairly low-stakes. It simply encourages students to reflect on the dynamics of agreement and disagreement. Even if students ultimately decide that the algorithm is misreading a conversation, it still will have accomplished its purpose. These kinds of analytics can be used in a synchronous discussion, an asynchronous discussion forum, or a social text annotation tool.
In fact, one of the most promising aspects of collaborative feedback EdTech affordances is their potential to become ubiquitous. They can be embedded in many different educational tools to help teach many different subjects and disciplines. All technology-enabled classes could include affordances that make facilitating rich and productive class conversations easier. As a result, instructors could be more likely to incorporate such discussions into their course designs more frequently.
Peer assessment
Once students are proficient in participating in collaborative feedback, they are ready to start learning peer assessment skills. To restate my earlier point, this is the milestone at which social learning pedagogies can begin to scale effectively. Why has EdTech pushed so hard on machine assessment? Because providing students with frequent, high-quality feedback is the bottleneck to scaling classes while maintaining quality. Machines have proven excellent at providing frequent and timely feedback to students, but often at the expense of quality. If students can be taught how to provide high-quality feedback to each other, then they can solve the assessment bottleneck more effectively than the machines can. Even better, the students will be learning essential workplace and life skills in the process.
To be clear, the path that I’m proposing is hard. Every academic knows from direct experience that even people with PhDs are not reliably capable of providing good peer review (or receiving it, for that matter). Anyone who has tried to implement peer assessment in even a small classroom knows that it is a lot harder to pull off than giving a decent lecture. Going back to the Agile example, software development shops that transition to Agile methodologies usually need a couple of years and some active, persistent coaching from an expert to become proficient at it.
To deal with this problem, a substantial majority of the rituals and artifacts in Agile methodologies are designed to improve these skills in the group. To cite another example, Agile teams will often “play the cards” at the beginning of each development sprint. A key part of reliable software development is accurately estimating how much effort—and therefore time—will be required to develop a feature. So at the beginning of a sprint, all team members may be given a set of cards representing different estimates for the amount of effort they think each feature will require. For each feature, the team members literally lay their cards on the table, comparing how much effort each person thinks a given feature will require. The goal is remarkably similar to the “thought distance” graph above. The team is trying to figure out the level of agreement about how much work is required. Each team member then has to defend her estimate. The team debates the merits of the various opinions and strives to arrive at a consensus estimate.
We do have EdTech tools that facilitate, scaffold, and scale peer assessment. There is some evidence that they can be effective, although I would like to see more research in this area. One of the (relatively) better-known products in this category is Peerceptiv. It can help organize students into groups, scaffold their peer evaluation work, and then scaffold peer evaluation of how supportive and effective their peers were during the exercise.
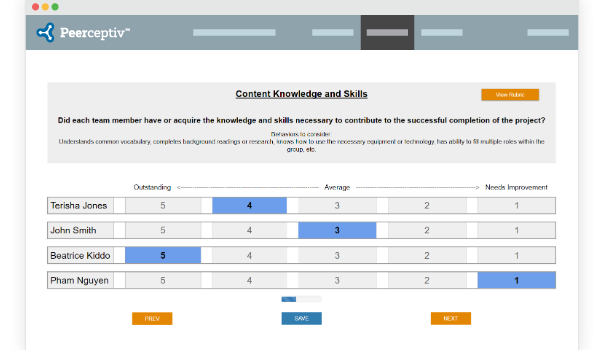
Peer assessment can be enhanced using a number of techniques. The simplest one is to assign a small but meaningful percentage of students’ grades based on the feedback quality scores that their peers give them. In other words, reward students for giving feedback that their peers find useful. Second, the instructor can selectively hop from group to group and act as a participant in some peer reviews (particularly if these reviews are asynchronous). She doesn’t have to do this with every group every time. But having an instructor present and modeling quality peer evaluation from time to time helps a lot. She’s essentially acting like a coach, moving from team to team. And finally, we can some of the same technological aids that I described in the previous section to continue acting as supplemental “guides on the side.”
One of the harder parts of this approach is designing good rubrics that students can be taught to apply consistently and effectively. The upside is that, if they are designed well, they can often be reused in various contexts. For example, I can imagine a shortlist of writing evaluation criteria that are included in every rubric template. To the degree that non-writing instructors adopt the peer evaluation tool and template, they will automatically implement a basic writing-across-the-curriculum program. Even if they are uncomfortable evaluating students’ writing, the students themselves will coach each other.
Making the transition
My proposal is ambitious. It likely will work best when applied consistently in different courses across different programs. Faculty would have to redesign their classes and think differently about their pedagogical approaches. Much of the technology required may exist but has yet to be stitched together into a coherent end-to-end experience that supports this approach. And we don’t have a lot of research on how well these strategies will work, especially in concert in a technology-enabled context.
But the raw materials exist. Research on the effectiveness of collaborative learning exists. Various technologies that could scaffold, support, and scale the pedagogy exist. And I reject the notion that instructors or students would not be willing to put in the work required en masse. The truth is that a discussion-based seminar in which ideas flow freely is almost the Platonic ideal of education for educators and students alike. A good seminar is a joy for everyone involved. We should not abandon the idea that we can provide this kind of opportunity for all students. Nor should we shirk our responsibility to teach students the skills required to participate in such learning experiences as an essential part of a college education.
Nothing I have written here should be read as a blanket repudiation of machine assessment or EdTech that focuses on helping students with self-study. Those approaches are necessary for scaling educational access with quality.
But they are not sufficient.
The post The Anatomy of a Scaled Digital Seminar appeared first on e-Literate.