AI, Cheating, and the Future of Work
e-Literate 2021-11-11
The Times Higher Education (THE) is out with a piece titled “Does AI Spell the End of Education?” The promotional blurb explains further,
Artificial intelligence will soon be able to research and write essays as well as humans can. So will genuine education be swept away by a tidal wave of cheating – or is AI just another technical aid that teaching and assessment will evolve to take account of? John Ross reports[.]
Does AI Spell the End of Education?
This is an excellent article. I don’t mean that it is insightful or well-written. While it has its moments, overall, it’s an unenlightening mess wrapped in clickbait packaging. It is not good writing or good journalism.
But it is a near-perfect illustration of how the popular representations of both artificial intelligence (AI) and cheating can be harmful.1
It also shows a way for educators to understand AI better because AI and cheating sometimes work in similar ways. I will explain the parallel in this blog post. In the process, I will also argue that framing cheating in the context of “academic integrity” is harmful. And I will argue that all of these misunderstandings are counterproductive to preparing students for the future of work.
People who cheat are not “cheaters”
As you’ve probably figured out by now, I’m going to treat the THE article harshly. I’ll try my best to avoid the oh-so-tempting cheap shots. (The original working title for my post was “Does AI Spell the End of Education Journalism?”) The deeper problem at the heart of this article deserves serious treatment. I’m going to argue that “Does AI Spell the End of Education” is an example of journalistic “cheating.” In the process, I’m going to take a somewhat unconventional position on what it means to “cheat.” That position is relevant not only to how AI is used in the classroom but also to how we should think about AI and knowledge work and to how we should think about so-called “academic integrity.”
As part of that reframing, I want to be very careful to separate judgments about the writing from ones about the article’s writer, John Ross. I don’t know the man. I also don’t know the assignment he was given that led to him producing this article. I have no opinion of him as a writer or a human being. I only have opinions about the quality of this piece and the writing process that led to it.
I define “cheating” as “engaging in behaviors that are intended to facilitate passing without learning.” This definition avoids passing a blanket judgment on the person engaging in the behavior. It doesn’t accuse them of lacking “academic integrity.” It simply identifies behaviors that facilitate students getting good grades—which in the workplace we might call “scoring well on key performance indicators (KPIs)—without actually doing the hard thought work necessary to complete the assignment as intended. Any scoring system can be gamed. People game scoring systems for all kinds of reasons. One might be pressure. Perhaps a student wants to learn but needs to pass. Or a journalist wants to write an insightful piece but needs to complete a hugely ambitious assignment with an unrealistic deadline or word count limit. Sometimes we engage in sloppy or lazy shortcuts not because we are sloppy or lazy people but because we feel forced to do so by the circumstances. Whether in the classroom or the workplace, our primary focus should be on reducing the incentives to game the scoring system rather than on punishing “cheaters” for their lack of “integrity.”
From here forward, I will distinguish between John Ross, the human author of “Does AI Spell the End of Education?”, and the mental algorithm he employed to write this piece, which I will call Journobot 2000. These two are not the same. John Ross may very well be a smart guy. Journobot 2000 is a set of mental shortcuts that John Ross employed to avoid the hard work of thinking and learning when writing parts of his article. It does not understand AI, cheating, or the teaching of writing. It is capable of assembling passages about such topics in ways that sound coherent. It can even fool some intelligent readers into thinking that its output reflects some understanding of these topics. But Journobot 2000 does not understand anything. It is simply a sophisticated pattern-matching algorithm that can copy/paste in interesting ways and employs a souped-up thesaurus to rephrase sentences.
Journobot 2000 is a cheating strategy. It enables a writer under pressure to produce an article that sounds coherent without forcing that writer to invest the time necessary to understand the subject. When students employ Journobot 2000—which many do—they do not learn. When knowledge workers do the same, they do not perform useful knowledge work.
Knowledge work and learning are the same. Knowledge workers solve novel problems. How do they do that? By learning. Learning, in turn, requires thinking. Shortcuts that reduce drudge work are fine, but ones that reduce thought work are dangerous if your work requires you to think and learn.
Writing as collage
Journobot 2000 has assembled a series of quotes and facts related to the topics of AI, writing, and/or cheating in some combination. Before we analyze how it does this, let’s look at a few of the individual quotes from interviewees that appear in the article. I’ve arranged these out of order from their placement in the article for a specific reason. Think about each of these passages on its own and consider which issue or issues each speaker is concerned about.
I’ll provide fairly extensive quotes from every person to provide the flavor of their concerns. The first passage quotes Lucinda McKnight, a senior lecturer in pedagogy and curriculum at Deakin University:
“How do we prepare teachers to teach the writers of the future when we’ve got this enormous fourth industrial revolution happening out there that schools – and even, to some extent, universities – seem quite insulated from?” McKnight asks. “I was just astonished that there was such an enormous gap between [universities’] concept of digital writing in education and what’s actually happening out there in industry, in journalism, business reports, blog posts – all kinds of web content. AI is taking over in those areas.”
McKnight says AI has “tremendous capacity to augment human capabilities – writing in multiple languages; writing search engine-optimised text really fast; doing all sorts of things that humans would take much longer to do and could not do as thoroughly. It’s a whole new frontier of things to discover.”
Moreover, that future is already arriving. “There are really exciting things that people are already doing with AI in creative fields, in literature, in art,” she says. “Human beings [are] so curious: we will exploit these things and explore them for their potential. The question for us as educators is how we are going to support students to use AI in strategic and effective ways, to be better writers.”
And while the plagiarism detection companies are looking for more sophisticated ways to “catch” erring students, she believes that they are also interested in supporting a culture of academic integrity. “That’s what we’re all interested in,” she says. “Just like calculators, just like spell check, just like grammar check, this [technology] will become naturalised in the practice of writing…We need to think more strategically about the future of writing as working collaboratively with AI – not a sort of witch-hunt, punishing people for using it.”
Does AI Spell the End of Education?
That’s interesting. I agree with some of McKnight’s comments and have questions about others. For example, there’s an enormous difference between writing search-engine-optimized (SEO) text really fast and writing informative and well-written SEO text really fast. What is the relationship between the tool and the knowledge worker here? I have an SEO tool in my blog. It hates my writing. The feeling is mutual. If I followed its recommendations slavishly, I would have many more people coming to my site and many fewer reading it.
For now, the takeaway is that McKnight is interested in teaching students about how they might use AI text generation tools in the workplace. Let’s save further exploration of this line of thinking for later in this piece.
The next person in the article whose concerns I’d like to explore is Dr. Jesse Stommel, Digital Learning Fellow and Senior Lecturer of Communication and Digital Studies at the University of Mary Washington. Stommel is concerned about anti-plagiarism software. Here is how he is quoted:
“They have data about student writing,” he says. “They have data about how student writing changes over time because they have multiple submissions over the course of a career from an individual student. They have data where they can compare students against one another and compare students at different institutions.”
The next step, Stommel argues, is the development of an algorithm that can capture “who my students are, how they grow, if they’re likely to cheat. It’s like some dystopic future that is scarily plausible, where instead of catching cheaters, you are suddenly trying to catch the idea of cheating. What if we just created an algorithm that can predict when and how and where students might plagiarise, and we intercede before they do it? If you’ve seen Minority Report or read Nineteen Eighty-Four or watched Metropolis, you can see the dystopic place that this will ultimately go.”
Does AI Spell the End of Education?
Stommel is focused here on student data privacy, which can be a critical issue of certain applications of both AI and non-AI EdTech. While I don’t agree with his assessment regarding the plausibility of his nightmare scenario, I completely agree with the concern he is highlighting and would like to see it unpacked and explored. I could easily write an entire long post explaining which fears are realistic and why or why not. Notice, though, the concern Stommel expresses here isn’t about text generation tools or even AI specifically.
The third quote from the article that I’d like to highlight is from Andrew Grauer, CEO of Course Hero. He said,
“I’ve got a blinking cursor on my word processor. What a stressful, inefficient state to be in!” he says. Instead, he could use an AI bot to “come up with some kind of thesis statement; generate some target topic sentences; [weigh up] evidence for a pro and counter-argument. Eventually, I’m getting down to grammar checking. I could start to facilitate my argumentative paper.”
Does AI Spell the End of Education?
This, too, is interesting and worth exploring. When is this sort of support scaffolding that helps students learn, and when is it a crutch that helps them avoid learning? I did write about this topic as part of a larger post on scaling the digital seminar and could easily write more about it.
Grauer’s quote does seem related to McKnight’s. They’re both interested in how AI can scaffold writing. When John Ross interviewed people for the article that would eventually be named “Does AI Spell the End of Education?”, he did seem to probe his interviewees to foster a genuine dialog on this aspect of the article. He even introduces a quote from Turnitin’s Chief Product Officer Valerie Scheiner that acts as connective tissue between the two others. Here’s her relevant passage:
Turnitin is now using AI to give students direct feedback through a tool called “Draft Coach”, which helps them avoid unintentional plagiarism. “‘You have an uncited section of your paper. You need to fix it up before you turn it in as a final submission. You have too much similarity [with a] piece on Wikipedia.’ That type of similarity detection and citation assistance leverages AI directly on behalf of the student,” [Scheiner] says.
But the drawing of lines is only going to get more difficult, she adds: “It will always be wrong to pay someone to write your essay. But [with] AI-written materials, I think there’s a little more greyness. At what point or at what levels of education does using AI tools to help with your writing become more analogous to the use of a calculator? We don’t allow grade-three students to use a calculator on their math exam, because it would mean they don’t know how to do those fundamental calculations that we think are important. But we let calculus students use a calculator because they’re presumed to know how to do those basic math things.”
Schreiner says it is up to the academic community, rather than tech firms, to determine when students’ use of AI tools is appropriate. Such use may be permissible if the rules explicitly allow for it, or if students acknowledge it.
Does AI Spell the End of Education?
This seems to be a direct response to McKnight’s quote while nodding at some of the ethical issues raised elsewhere the piece. The most interesting part of “Does AI Spell the End of Education?” is the tension—and arms race—between text generation tools and plagiarism detection tools.
But the piece never quite manages to fully focus on this dilemma. It’s weirdly fragmented. There’s a one-sentence reference to “word spinners,” which are text paraphrasers that can be used to disguise plagiarism. But Ross never follows up on this angle, despite the fact that it fits perfectly with the dialog on text generation he’s assembled with the quotes from McKnight, Grauer, and Scheiner. Instead, he just supplements that one-sentence mention with a link to an article about word spinners on Turnitin’s web site. And then there’s Stommel’s quote, which is stuck in the middle of the piece and doesn’t seem directly related to the rest of the narrative. Student data privacy is not raised either before or after. The quote is just…there.
Why?
The answer is that John Ross, the human writer, cheated. This article seems like the result of a reporter who has interviewed a range of experts on the topic of AI in education as part of an effort to understand and report on the issues.
But it isn’t.
Several interviewees told me that they were interviewed months ago on topics other than AI and the teaching of writing. One of them, Jesse Stommel, went on record for me on this topic. He told me that he was originally interviewed about Turnitin’s acquisition of one of its competitors. While he does not object to authors using his quotes in other articles, he said, “[M]y quotes were not direct reflections on AI.” In fact, AI did not even come up in his interview.
When read with this in mind, the article makes much more sense. The most coherent parts of the writing were on threads that would have fit in the context of an article on Turnitin and anti-plagiarism software. The parts that get messy are precisely those where John Ross’s original research on a Turnitin story did not line up well with the purported topic of the article. For example, Stommel’s quote would have fit more naturally in the anti-plagiarism software piece because he was voicing concern about how anti-plagiarism software uses student data.
When John Ross decided to use some of the material from his original, never-published piece on Turnitin, he could have gone back to Stommel and asked him for questions that would have been directly relevant to the AI article. But he didn’t. Why not? I don’t know. Maybe he was lazy. Maybe he was under time pressure. Maybe his editors wanted something particular from him. I’m not going to judge the human being based on one article.
But I am going to judge his work on the article itself. For whatever reason, Ross fired up Journobot 2000. Rather than conducting further research, he took what he had already from a piece on another topic. He rearranged the pieces to look like they had always been intended to be parts of an article on AI. Journobot did so by following a simple pattern that I’ll analyze in the next section.
This is remarkably like the strategy students take of plagiarizing an essay on a similar topic to the one they’ve been assigned and then rearranging it to try and make it fit. The only difference is that he was plagiarizing himself. The problem here isn’t taking somebody else’s thoughts and claiming them as your own. It’s claiming to have thought about and analyzed a topic when you haven’t.
When students do this sort of thing, we call it “cheating.” It results in them failing to think and learn. When journalists do it, we call it “lazy journalism.” It results in messy articles that fail to enlighten the reader. More generally, when knowledge workers do it…well, we don’t have a specific name for it, but it results in low-quality work.
In data science, we call it “artificial intelligence.”
What cheating looks like
Journobot 2000 does not understand the relationship between Jesse Stommel’s data privacy concern and AI. It’s matching two kinds of patterns. First, since this is an article on a controversial topic, it represents controversy by alternating between quotes with positive sentiment scores and ones with negative sentiment scores. It’s simulating point/counterpoint. John Ross, the human journalist, could have chosen to leave out the hyperbolic end of Stommel’s quote and focused instead on the underlying concern. Journobot 2000 likely found that quote to fit its pattern-matching algorithm precisely because of the ending, which expresses a strong negative sentiment about something related to the topics at hand. It also knows how to write transitional phrases so that one passage appears related to the next.
Speaking of which, Journobot 2000 knows that anti-plagiarism software, AI, cheating, and writing are related topics. It organizes the quotes in ways that show relatedness among the topics. Because it doesn’t really understand the topics the same way humans do, a careful reader can see the seams where the piece doesn’t really hold together. But a casual reader might not notice that Stommel’s quotes have been spackled into places where they only loosely fit with the analysis that comes before or after. He’s not really part of the dialog in the same way that some of the others were.
Likewise, there’s that largely unutilized reference to word spinners. In an article about Turnitin, the topic might have only made sense to mention as one of many aspects concerning the company and its acquisition of a competitor. But in an article about AI potentially ending education, word spinners should have received significant attention. John Ross might have seen that and researched accordingly. Journobot 2000 did not make the connection.
Let’s pick up on a couple of the threads missed by Journobot 2000 to get a sense of the article that could have been if John Ross had applied the same level of attention that the archeological evidence in his published piece suggests he put into the original, unpublished version.
Articles written by actual machines
Let’s start with the wonders of machines writing articles. You have almost certainly read articles written by a machine. For example, if you follow stocks, you may have already learned to recognize the articles written by bots. Imagine a massive drop in the stock price of a biotech stock because they had bad clinical trial results. You might read a perfectly well-written financial news story in your inbox, telling you all about the technical indicators on the stock price, complete with a headline suggesting the article will provide insight as to whether to buy or sell…but no mention whatsoever of the news that drove the price move. The technical analysis is data-driven and seems perfectly cogent. The writing has just a dash of colorful language, suggesting the barest hint of a simulated authorial voice. If you didn’t know about the news, it would seem normal. But it’s not really a financial analysis news piece. It’s a data analytics report written in narrative form with a formulaic headline tacked on the top. The machine doesn’t really understand the topic it’s writing about.
In this example, there may be little to no actual artificial intelligence involved in the writing. A human might have written a template covering the topic of a certain type of stock movement. The software fills in the data. It has been provided with a handful of colorful phrases to substitute for different common phrases. “The stock took a nosedive.” “The stock tanked.” “The stock plummeted.” These can be interchanged randomly to create the appearance of an author behind the piece.
Genuine AI can generate original writing using a family of techniques called Natural Language Processing (NLP). A particular product called GPT-3 produced by a company called OpenAI is getting most of the buzz right now, but there are others. It can produce uncanny writing. By which I mean writing that falls in the uncanny valley. It’s writing that seems sort of human but not quite. The result is weird and sometimes creepy. (To get a delightful sense of just how weird and creepy, read Janelle Shane’s blog AI Weirdness. And then read her book, You Look Like a Thing and I Love You: How Artificial Intelligence Works and How It’s Making the World a Weirder Place.)
A recent article on NextWeb, “Don’t mistake OpenAI Codex for a programmer,” is illustrative. It’s all about how the Microsoft-owned Github software repository platform took a highly customized version of GPT-3 and trained it to write computer code. The idea is that if GPT-3 can learn English, then it should be able to learn Javascript. Programming languages are languages, after all.
A good part of the article is devoted to the No Free Lunch Problem, “which means that generalization comes at the cost of performance. In other words, machine learning models are more accurate when they are designed to solve one specific problem. On the other hand, when their problem domain is broadened, their performance decreases.” Even an enormous, computationally expensive, state-of-the-art AI program like GPT-3 is mediocre at performing a wide range of tasks. Developers invest enormous time and energy tuning it to do one thing really well. And even then, “really well” isn’t always…um…all that well. Here’s the money quote from the piece:
In their paper, the OpenAI scientists acknowledge that Codex “does not sample efficient to train” and that “even seasoned developers do not encounter anywhere near this amount of code over their careers.”
They further add that “a strong student who completes an introductory computer science course is expected to be able to solve a larger fraction of problems than Codex-12B.”
Don’t mistake OpenAI Codex for a programmer
While I don’t know how much money Microsoft spent on developing Codex, I’m confident it cost at least several orders of magnitude than the typical EdTech AI. And yet, it can’t match a first-year computer science undergraduate.
Why not? The piece goes into some technical detail, but it boils down to the fact that today’s AI still has some sharp limitations relative to humans when it comes to problem-solving. It can’t hold as many relevant facts in its “head” as we can. It doesn’t match patterns in the same way. It’s not as good at catching nuances of meaning in language and relationships among ideas. While the progress being made in AI today is miraculous, it’s not biblically so. It’s not magic. If one of the most expensive and technologically advanced algorithms in human history can’t match a first-year college student, then we should probably let go of the breathless hyperbole about AI “ending education” for a while.
Rather than employing Journobot 2000, John Ross could have engaged his full human faculties as a learner, thinker, and knowledge worker to engage with the purported topic of his article. He has many of the raw ingredients for something genuinely interesting. But he didn’t take the time to follow the threads.
Word spinners are another example.
Spinning words
John Ross’s article mentions “word spinners”—tools that rewrite sentences using AI—as cheating tools to get around plagiarism detectors. But it doesn’t name any or explore the topic in detail. The most he does is link to an article about word spinners on Turnitin’s website (which is probably another artifact of the original article).
In the absence of John Ross’s due diligence, I conducted a little of my own by employing an advanced AI research tool called Google. It turns out not all word spinners are the same. For example, Rewriter Tools Article Spinner all but explicitly advertises itself as a tool that is designed for cheating:
Today, almost everything is done online – including work assignments, student essays, and anything else you can think of. As a result, a large amount of written work also has to be done online.
The problem is that so much has already been written about pretty much everything, that creating completely new and unique content is quite difficult. Not to forget, also time-consuming and rather tiring, too. As a result, many people get confused and frustrated while trying to create unique content.
Do you want to create original, fresh content but are pressed for time? Rewriting a document to make it unique is not always an easy task. This is why we present you with Article Spinner – the perfect to help you create fresh content in very little time.
Probably some bot
Ladies and gentlemen, welcome to the future of knowledge work! Papers that are badly rewritten by a tool created by a bad writer because thinking is too hard and who has original ideas anymore anyway?
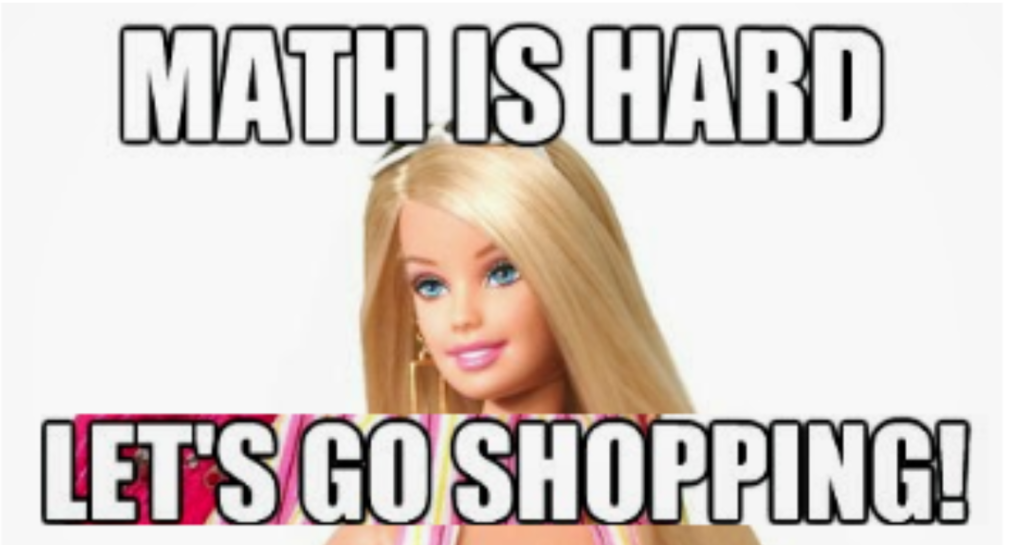
On the bright side, their search engine optimization algorithm must be good because this text put them near the top of my search results page.
Quillbot, on the other hand, positions itself as a tool that helps writers tune their language to their audience:
Your words matter, and our paraphrasing tool is designed to ensure you use the right ones. With 3 free modes and 4 premium modes to choose from, QuillBot’s paraphraser can rephrase any text in a variety of different ways, guaranteeing you find the perfect language, tone, and style for any occasion. Just enter your text into the input box, and our AI will work with you to build the best paraphrase from the original piece of writing.
A slightly more sophisticated bot
Is that better than Article Spinner? I think it may be worse. First, it appears to be more sophisticated at rephrasing other people’s work. When McKnight talks about the Fourth Industrial Revolution and AI helping humans do their jobs better, I don’t think she means AI helping college students take pieces written by somebody else and paraphrasing them in varied ways to pass a plagiarism detector.
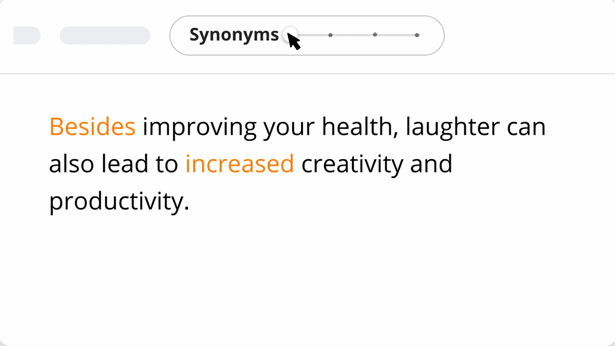
Second, again, I’m having a hard time coming up with legitimate use cases that aren’t just shortcuts to avoid thinking. I use a grammar checker that makes style suggestions—more on that momentarily—but it doesn’t wholesale rewrite for me. Instead, it highlights choices that I can make as a knowledge worker. Quillbot calls itself a “paraphraser.” (Side note: Judging from the text on both sites, I’m guessing that “paraphrase” may be a good SEO term for both products.) Maybe there are some legitimate uses for a tool that can quickly paraphrase a longer document. If I write a follow-up post to this one, I may try using it on a previous post to see if anything useful comes out.
Then there are grammar checkers, which are mentioned but—again—never explored in “Does AI Spell the End of Education?” I use Grammarly Premium regularly. In fact, I am using it right now. It helps me catch mistakes and write clearer, punchier prose. Even though I am a pretty good writer, Grammarly improves almost everything I write (when I use it). But it is only useful to me because I know when—and why—I should ignore or overrule its suggestions. If I were to ask students in a writing class to use it, I would have to teach them to do the same. The problem is that I don’t know how Grammarly works. I can’t teach students how to anticipate all the mistakes it might make.
This is particularly true with students who have language patterns that Grammarly might not anticipate. For example, second-language learners whose native language is Chinese or Russian may write English sentences that drop certain types of words (like articles or pronouns), mix up verb tenses, mess up idiomatic expressions, and change the word order. And even fluent second-language learners may make mistakes that the grammar checker won’t diagnose correctly when the writers are stressed, such as when they are trying to express difficult ideas while writing under time pressure. In combination, these problems could confuse a grammar checker and cause it to make a bad suggestion.
As a result, I would have to think hard about whether, when, and how to use Grammarly as a teaching tool, even if I believed it would help most students improve their writing the majority of the time. As a writing teacher, my job isn’t to get students to produce better writing. It’s to teach them how to be better writers. As a writer, while I use Grammarly to help me edit my text more quickly and effectively, I also use it to help me make mindful decisions about when to break the rules. Good writers balance clarity against expressiveness all the time. Sometimes I override Grammarly not because its suggestion is wrong but because I have chosen to write a more challenging sentence to read to communicate a challenging idea more effectively.
I would have liked to read a researched article on this topic. I suspect John Ross could have written it. Journobot 2000 cannot.
The bottom line
The future of work is knowledge work. Knowledge work and learning are the same. Therefore, if we want to prepare students for the future of work, we need to teach them how to think and learn. Cheating is behavior intended to achieve a passing grade without learning. Cheating is bad because it leaves students ill-prepared for the future of work (not to mention for life). Tools or strategies that help knowledge workers (including students) avoid mindless work are probably good more often than not. Tools or strategies that help knowledge workers avoid thought work are probably bad. More often than not.
“Does AI Spell the End of Education?” raised (but did not explore) authentic assessment as one way out of the cheating problem. While I’m a fan of authentic assessment, the article itself is proof that it is not a panacea. Because it is, in fact, an authentic assessment of John Ross’s writing. As a writing portfolio artifact, the piece shows that the author could pass, i.e., get his article published, without learning anything new about the promise and perils of AI in education.
Many decent educators have faced the challenge of trying to break students out of algorithmic behaviors that have enabled them to pass without learning, whether the behavior is writing a robotic five-paragraph essay or memorizing physics equations without understanding them. If cheating is the set of behaviors designed to succeed without learning, then these behaviors, which have been taught to students as perfectly appropriate, are cheating just as much as copying somebody else’s answer is. It matters in the classroom, it matters in the workplace, it matters in the home, and it matters in the ballot booth. I hope the next article I read about AI and cheating will be about applying AI to solve that problem.
- The THE article also completely elides the difference between artificial intelligence (AI) and its cousin machine learning (ML). This is forgivable because the reader doesn’t need to understand the difference for the purpose of the piece. I’m not going to delve into the distinction in this blog post for the same reason. But I’m aware there is one. When I refer to AI, please read that as shorthand for the larger family of AI and ML techniques.
The post AI, Cheating, and the Future of Work appeared first on e-Literate.